SDO 014 - Rethinking Your Data Strategy - Vin Vashishta
Interview: Vin Vashishta, Founder & Technical Strategy Advisor at V Squared
What are your thoughts on strategy?
Data leaders often talk about the importance of strategy, yet many fall for the trap of confusing strategy with tactics. The three guiding questions that help me maintain a strategic mindset are the following:
How can data create a competitive advantage in the market, where the result of the initiative enables a clear differentiation from competitors?
What are the high-touch human tasks that drive revenue (e.g., enterprise sales), and how can data optimize the key points leading up to these high-touch tasks?
What assumptions drive my strategy, and how can I incrementally validate them to reduce the risk of implementing one strategy over another?
These questions were formed through my numerous conversations with Vin over the past few years via Zoom office hours, strategy courses taught by Vin, and even one-on-one career coaching sessions I have had with him. Even with all of these conversations with Vin, I still feel like I’m only scratching the surface of his knowledge on the subject— hence why I’m so excited for you to read my interview with him below. Enjoy!
— Mark
Hear from Vin Vashishta, Founder & Technical Strategy Advisor at V Squared:
Vin ranks as one of the top three people that has helped elevate my data career with his wisdom. I specifically attribute his data and AI strategy course to helping me get promoted to a senior data scientist and driving tremendous value at my job. In addition, I used to have near-weekly conversations with him on the Artist of Data Science Happy Hours, where he would drop gem after gem of knowledge, which slowly pushed me to be a more strategic thinker. In short, I’m beyond excited for you all read the below insights from Vin!
One of my biggest takeaways from our previous conversations is the need for data teams to be positioned as strategic partners rather than cost centers. What do you believe is necessary for a data team to be positioned as a strategic partner?
Vin: “We have to have revenue booked against our names. If cost savings are great, productivity is great. That's gonna get buy-in. That's sometimes what you're stuck with in the early days. But if we don't have revenue, there's no point to our existence because we're too expensive.
Software development teams are the paradigm companies are used to. That's the cost structure they're used to. When they start digging under the covers and CFOs are, believe me, they're lifting up the curtain right now and they're looking behind and seeing what's going on. They realize how expensive in comparison to software engineering that data teams are.
It's the people that are more expensive. It's the infrastructure that needs to be built out that's more expensive. Data gathering just by itself is more expensive. We're going to quickly shift from data gathering to data curation, but only after, as a data science team, we start showing a value proposition that grows the business. But that's the interesting piece of where we're going.
Companies are going to start seeing because now they're forced to monetize data. Startups need a path to profitability. That's gonna be one driver for this trend. Companies that are struggling to remain competitive need new growth areas; they need to expand into new marketplaces. So in big tech, those companies that are struggling for growth, to maintain their share price and maintain their multiples, they need ways to grow. They're going to turn to data. It's gonna be another driver of this.
And in traditional legacy businesses, traditional industries, those companies have to figure out how to maintain margins in the face of the current inflationary cycle. But longer term, they're now challenged by tech companies who are going to come into their industry and begin to compete with them on best in class technical capabilities.
So those are your main drivers of what comes next. Data teams either step up or their businesses are going to fail. So you'll see these use cases over the next year start emerging more and more where a company that made maybe 40 million, 50 million in total revenue in 2022 figures out that they have a data gold mine. And by the time 2024 comes around, they're in 500 to a billion. Because that's the power of data. It is truly insane how much money you can make off of a few initiatives. But you have to do things in a more strategic way. The data team needs to put itself at the strategy planning level in order for opportunity discovery to be done properly.
We have to have an entirely different conversation with the C-Suite to explain how data creates value differently than digital products create value. There's a different life cycle, a different process for management. It's going to take a company that's desperate. Or a company that realizes the absolute just blue ocean gold mine that's in front of them.
If C-Levels realize that, the company will quickly grow and it'll be one of those early use cases. If it doesn't, it will not survive. So that's going to drive this trend over the next 12 months is this realization of risk and peril as well as opportunity and what it will take. But once we get a few of those use cases out in public, it will be a land grab for data.
Dive deeper into this response with the following suggested reading from Vin:
You have recently highlighted the importance of data product managers for enabling high ROI data initiatives. Why should leaders start paying more attention to this role?
Vin: “First, we have to realize that C-Level doesn't understand how to monetize this. So they're doing a traditional opportunity discovery process, which does not include data opportunities. They are looking at everything from a digital lens, if we're lucky. But more than that, we're also getting more of a legacy lens brought to modern technical strategy.
So that's driver one, is the C-Level isn't doing opportunity discovery with a full picture of what's out there that's threats and opportunities. We're also not exploiting the opportunities available to us in our existing product lines. So we need to have this top down and bottom up opportunity discovery. Top down is very focused on TAM, your total market, that big, big picture. Bottom up is focused on your SAM and your SOM.
How much of that market can we capture and how much can we service? Now you're hearing efficiency projects, you're hearing new features for existing product lines. How do we extend the lifespan of existing products using data, leveraging new features? How do we get technology introduced into workflows. Once we do that, we have the opportunity to deliver data, do experiments, provide functionality to customers.
That's what needs to happen, and a data product manager does that. That's lesson one and two in my class, it's just that. Because it's so high value, as soon as you get that perception change driven at the top and the bottom. It's the beginning of data literacy, but really moving towards what's model literacy, and that's our next step.
We can't just make people at every part of the business understand, okay, data is this, but it's models. Models are different. They don't work the same as digital products. We have to start making the organization making the entire enterprise more model literate. That means they'll be able to articulate their needs better.
That happens at the C-Level explaining we have these strategic goals, here are our needs, what initiatives could meet those needs? Data product managers can help. Frontline, here are the opportunities we are seeing here are the underserved customer segments ,and even internally, here are the underserved user segments.
Data product managers are out there finding these opportunities. What's happening right now is data science teams have to do all of this. It is rare to find someone who can do that on your data science team. It is a completely different capability set and you rarely have someone who has that combination of technical and strategy who can play this role?
So we're putting people, sort of forcing people into service who are not in the right capabilities framework to do this. They're set up to fail. It's also asking a whole lot because what you have to do in order to get these early initiatives out the door doesn't scale. You have to spend so much time with customers, so much time with your front end users, so much time with your C-Level.
That as soon as it flips and you start saying, okay, we're gonna scale and we're going to handle more use cases, we're going to be doing more initiatives, you suddenly hit this massive roadblock because you don't have anyone to take on this work. The data team is asked to do their job and play a strategy role and play a product facing role and manage stakeholders, and you can't do that.
You just can't ask your data team to do that without introducing another role. So that's why data product managers are critical, and by extension that's why technical strategists are critical. That's why strategic leaders at the organizational level, running data teams are critical. Bringing in a CDO or a CDAO early is not a bad idea. Having them be able to take the entire team, just process end-to-end, growing the business around data, it's a different ecosystem and you need new roles.”
Dive deeper into this response with the following suggested reading from Vin:
Given the changing market, what advice would you give leaders in rethinking their data strategy in a world where capital is expensive again?
Vin: “It has to be a sustainable business model. We've forgotten what that looks like. It needs to focus on-- I say this a lot-- great data products don't start with technology, great data products start with users and customers. It starts with their needs. You find an unmet or underserved customer segment, and that's where you start data products and you work your way back, find use cases and needs that cannot be met any other way.
That's what changes, is we have to do this in a more rigorous way. We have to create frameworks or it's chaos. We have to introduce some order to the process of selecting opportunities, defining initiatives, measuring success, prioritization frameworks are critical. We need self-service tools because data teams shouldn't be handling reporting requests.
We got infinite technology in the universe and we're still acting like we're driving around in a Ford POS. I mean, just it's so backwards right now. So we need to approach this from a completely different perspective, and that is monetization cash first. Not growth at all costs. Growth at all costs should be an organic thing.
Great products are going to grow organically as long as the company understands how to do basic sales and marketing organically. Look at OpenAI. That's the blueprint for how you get communities built and ecosystems built around products. Microsoft is teaching that masterclass right now on how to monetize models where people pay for inference, not for some feature you're building on top of it. They are paying for raw inference.
That's why OpenAI is such a valuable company right now is they're the first ones who have ever had a viable AI or machine learning as a service business model. It's the first one we've ever seen, which is viable. It's not BS. This isn't vaporware. It's actually able to power functionality and features people will pay for.
Once we get about six months to a year of track record, this will shift perception. Because it's real, and that's when CEOs start getting interested is when they start seeing cash, strategy comes forward. This is going to be the shift in thinking: how do we actually make money?
Now the CEOs see the technology is viable. Why? Because things are actually being delivered that are products that are working. They can see it work. They can put their hands on it. They can use DALL-E-2. They can use Stable Diffusion. They can use ChatGPT. They can use all this stuff. And for the first time, they know they're using a model supported product.
So CEOs are going to get very, very interested in anyone who can explain how to make this business model work. That's the opportunity for data scientists who are looking for a different place to go in their career. If you have that business side, you can be a strategic leader, you can be a data product manager, you can be a technical strategist.
You can go into one of those roles and help the business redefine how it functions. Redefining the business model to include a technology model, helps the company sort of frame this journey, this continuous transformation that they'll be going through. But if we don't connect it to value, there's no way to justify the cost.
That's the shift in thinking we have to create sustainable, viable business models. These have to pay for themselves incrementally. We can't say growth at all costs, it'll sort of maybe come together later. We have to have a definitive timeline for returns. We have to talk about break even. Those words have to come out of our vocabulary.
When will we break even? When will this initiative be profitable? How do we ensure that there is a high likelihood that we will deliver. And that we will get the returns that we're expecting. All of these are new concepts, strategies coming forward, monetization, customer needs. All of that is coming forward. Those are all the main changes that we're going to see coming in the next 12 months.
Some data teams will successfully navigate the new environment. They will thrive. You'll see investment in them, even in companies where revenues are declining. You'll see growing data teams because the monetization is there and it's proven. In other businesses, you're going to see the data team commoditized.
They will be smaller, they will be focused on some pretty rudimentary use cases, not going to be fun to work at those companies, and the demand for some roles will suffer significantly. Some data scientists are going to struggle to find a job because they're slowly realizing that what they've been doing for the last five years, seven years isn't really data science.
It's more what self-service tools now are starting to handle. Data scientist is gonna be challenged. This will be a challenging, but really opportunity filled time. So I don't mean to sound gloom and doom. This is a great time to be a data scientist. We just have to change a few things.”
Dive deeper into this response with the following suggested reading from Vin:
Person Profile:
Vin Vashishta is the Founder & Technical Strategy Advisor at V Squared. Feel free to connect with him on LinkedIn, Twitter, and his Substack
to learn more about his work.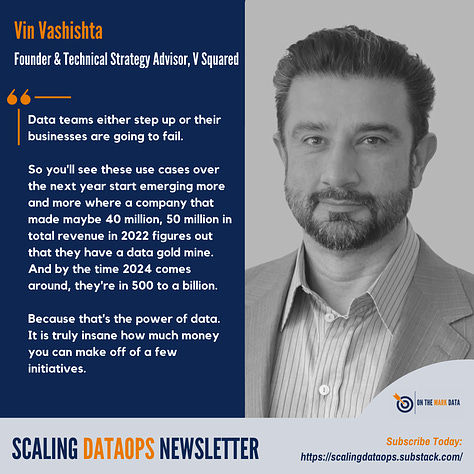
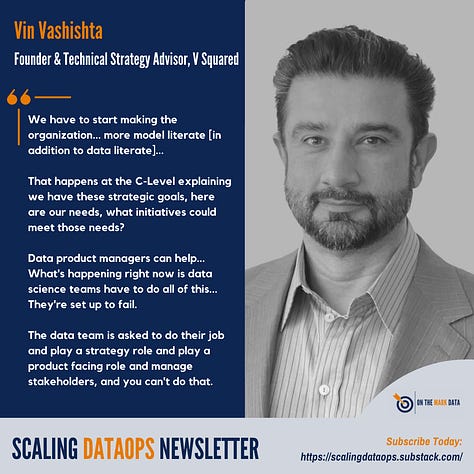
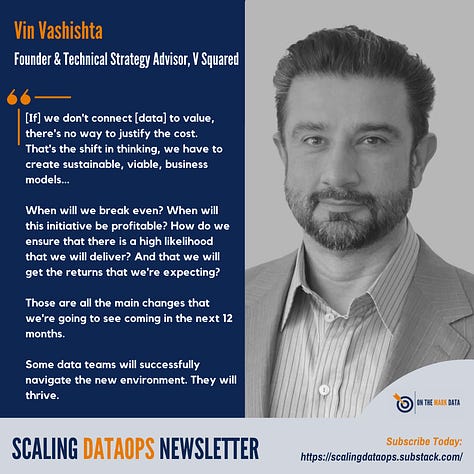
P.S. Are you looking for a community of data professionals actively growing their strategy skills? Then please check out my friend, Nicole Janeway Bills’s, community Data Strategy Professionals! I’ve gone to a few of her online networking events have met some awesome people that are also interested in strategy.